The Invited Talks at IDEAL 2024 bring together experts to share their latest research and practical insights straightforwardly and engagingly. These sessions are an excellent opportunity to explore new ideas, tackle current challenges, and see how cutting-edge techniques are applied in the real world. This year, we are very proud to host two invited talks:
Inverse Problems in the Age of AI
Inverse problems involve reconstructing unknown physical quantities from indirect measurements. They appear in various fields, including medical imaging (e.g., MRI, Ultrasound, CT), material sciences and molecular biology (e.g., electron microscopy), just to name a few examples. Deep neural networks are currently able to achieve state-of-the-art performance in many imaging tasks and in this talk we argue that in inverse imaging problems efficient deep neural networks with more predictable performances can only be achieved by combining model-based solvers with learned models. There is plenty of evidence for this and typical examples where this integration has had an impact include the plug-and-play framework and the network unfolding strategy.
In the first part of the talk, we introduce INDigo+, a novel INN-guided probabilistic diffusion algorithm for arbitrary image restoration tasks. INDigo+ combines the perfect reconstruction property of invertible neural networks (INNs) with the strong generative capabilities of pre-trained diffusion models. Specifically, we leverage the invertibility of the networks to condition the diffusion process and in this way we generate high quality restored images consistent with the measurements.
In the second part of the talk, we discuss the unfolding techniques which is an approach that allows embedding priors and models in the neural network architecture. In this context we discuss the problem of monitoring the dynamics of large populations of neurons over a large area of the brain. Light-field microscopy (LFM), a type of scanless microscopy, is a particularly attractive candidate for high-speed three-dimensional (3D) imaging which is needed for monitoring neural activity. We review fundamental aspects of LFM and then present computational methods for neuron localization and activity estimation from light-field data. Our approach is based on machine learning and leverages the intrinsic characteristics of neuronal signals and the physics of the acquisition process.
Finally, we look at the multi-modal case and present an application in art investigation. Often X-ray images of Old Master paintings contain information of the visible painting and of concealed sub-surface design, we therefore introduce a model-based neural network capable of separating from the “mixed X-ray” the X-ray image of the visible painting and the X-ray of the concealed design.
This is joint work with A. Foust, P. Song, C. Howe, H. Verinaz, J. Huang, Di You and Y. Su from Imperial College London, M. Rodrigues and W. Pu from University College London, I. Daubechies from Duke University and C. Higgitt and N. Daly from The National Gallery in London.
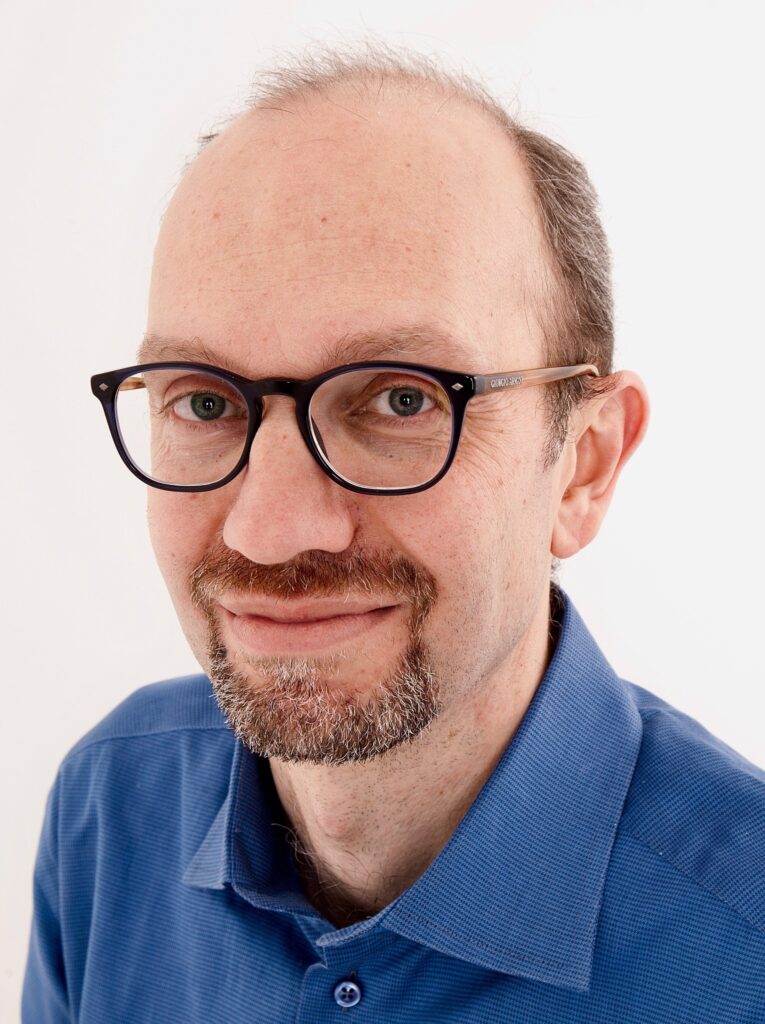
Pier Luigi Dragotti
Pier Luigi Dragotti is Professor of Signal Processing in the Electrical and Electronic Engineering Department at Imperial College London and a Fellow of the IEEE. He received the Masters Degree (summa cum laude) from the University Federico II, Naples, Italy, in 1997 and PhD degree from the Swiss Federal Institute of Technology of Lausanne (EPFL), Switzerland in 2002. He has held several visiting positions. In particular, he was a visiting student at Stanford University, Stanford, CA in 1996, a summer researcher in the Mathematics of Communications Department at Bell Labs, Lucent Technologies, Murray Hill, NJ in 2000, a visiting scientist at Massachusetts Institute of Technology (MIT) in 2011.
Dragotti was Editor-in-Chief of the IEEE Transactions on Signal Processing (2018-2020), Technical Co-Chair for the European Signal Processing Conference in 2012 and Associate Editor of the IEEE Transactions on Image Processing from 2006 to 2009. He was a SPS Distinguished Lecturer in 2021-22. He was also an Elected Member of the IEEE Image, Video and Multidimensional Signal Processing Technical Committee, IEEE Signal Processing Theory and Methods Technical Committee and of the IEEE Computational Imaging Technical Committee. In 2011 he was awarded the prestigious ERC starting investigator award (consolidator stream).
His research interests include sampling theory and its applications, computational imaging and model-based machine learning.
Bringing Deep Learning and Reasoning closer
Deep Learning continues to attract the attention and interest not only of the wider scientific community and industry, but also society and policy makers. However, the mainstream approach of end-to-end iterative training of a hyper-parametric, cumbersome, and opaque model architecture led some authors to brand it “black box”. Cases were reported when such models can give wrong predictions with high confidence – something that jeopardises the safety and trust. Deep Learning is focused on accuracy and overlooks explainability and the semantic meaning of the internal model representations, reasoning and its link with the problem domain. In fact, it shortcuts from the large amount of (labelled) data to the predictions bypassing and substituting the causality with correlation and error minimisation. It relies on assumptions about the data distributions that are often not satisfied and suffers from catastrophic forgetting when faced with continual and open set learning. Once trained, such models are inflexible to new knowledge. They are good only for what they were originally trained for. Indeed, the ability to detect unseen and unexpected and start learning this new class/es in real time with no or very little supervision (zero- or few- shot learning) is critically important but is still an open problem. The challenge is to fill the gap between the high levels of accuracy and the semantically meaningful solutions.
This talk will focus on “getting the best from both worlds”: the powerful latent feature spaces formed by pre-trained deep architectures such as transformers combined with the interpretable-by-design (in linguistic, visual, semantic, and similarity-based form) models. One can see this as a fully interpretable frontend and a powerful backend working in harmony. Examples will be demonstrated from the latest projects from the area of autonomous driving, Earth Observation, health and a set of well-known benchmarks.
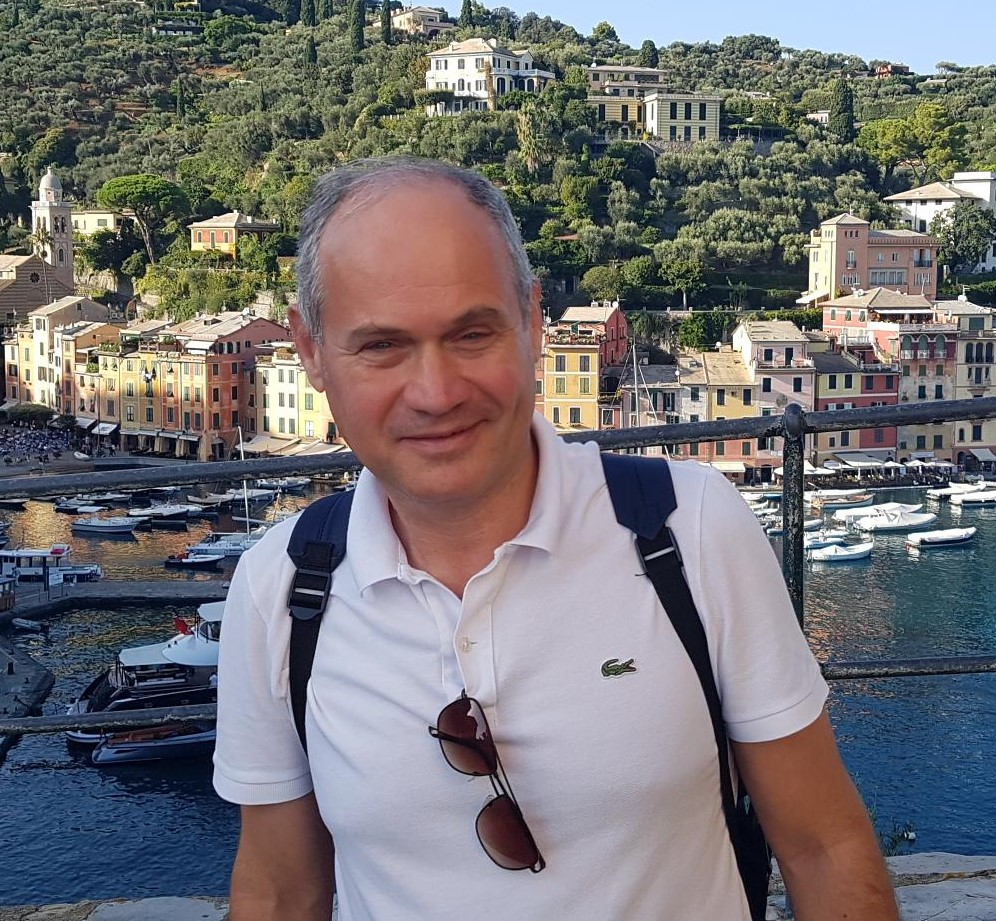
Plamen Angelov
Prof. Angelov (PhD 1993, DSc 2015) holds a Personal Chair in Intelligent Systems at Lancaster University and is a Fellow of the IEEE, IET, AAIA and of ELLIS. He is member-at-large of the Board of Governors (BoG) of the International Neural Networks Society (INNS) and of the Systems, Man and Cybernetics Society of the IEEE (SMC-S) as well as Program co-Director of the Human-Centered Machine Learning for ELLIS.
He has 400 publications in leading journals, peer-reviewed conference proceedings, 3 granted patents, 3 research monographs (published by Springer (2002 and 2018) and Wiley, 2012) cited over 15000 times (h-index 63). Prof. Angelov is has an active research portfolio in the area of explainable deep learning and its applications to autonomous driving, Earth Observation and pioneering results in online learning from streaming data and evolving systems. His research was recognised by multiple awards including 2020 Dennis Gabor award “for outstanding contributions to engineering applications of neural networks”. He is the founding co-Editor-in-Chief of Springer’s journal on Evolving Systems and Associate Editor of other leading scientific journals, including IEEE Transactions (IEEE-T) on Cybernetics, IEEE-T on Fuzzy Systems, IEEE-T on AI.
He gave over 30 keynote talks and co-organised and co-chaired over 30 IEEE conferences (including several IJCNN), workshops at CVPR, NeurIPS, ICCV, PerCom and other leading conferences. Prof Angelov chaired the Standards Committee of the Computational Intelligent Society of the IEEE initiating the IEEE standard on explainable AI (XAI). More details can be found at www.lancs.ac.uk/staff/angelov.
Human-Centered Artificial Intelligence: Challenges and Opportunities
HCAI has recently emerged as an important paradigm to design and evaluate AI systems. On one hand it is rooted in the heated debate about eXplainable AI (XAI), including interpretability, understandability as well as trustworthiness of AI. On another it also builds upon User-Centered Design techniques. HCAI puts emphasis on AI technologies that are built with humans and for humans thus enhancing human capabilities while ensuring systems are transparent, and under human control. The development of such technologies needs to unite a wide range of stakeholders, including from researchers, and developers, through policymakers to end-users. In HCAI, it is common to include social scientists working closely with engineers and designing empirical studies. The goal is to encourage a comprehensive discourse on creating AI systems that are tuned to user needs and consider user interactions, experiences with AI, and finally system assessment from the user perspective. In this talk we will give an overview of the diverse HCAI field and discuss examples of different HCAI research activities.

Grzegorz J. Nalepa
Grzegorz J. Nalepa (GJN.re) is a full professor at the Jagiellonian University, in Krakow, Poland. In 2024 he joined the ELLIIT excellence center and Halmstad University in Sweden as professor in machine learning.
He is an engineer with degrees in computer science – artificial intelligence, and philosophy. He also works as an independent expert and consultant in the area of AI (KnowAI.eu). He coordinates the GEIST.re research group in AI.
He co-authored over two hundred research papers in international conferences and journals. He has been involved in tens of projects, including R+D projects with number of companies. He authored a book “Modeling with Rules using Semantic Knowledge Engineering” (Springer 2018). In 2020 he founded to Jagiellonian Human-Centered AI Laboratory (JAHCAI), now part of the Mark Kac Center for Complex Systems Research.
His recent interests include applications of AI in Industry 4.0 and business, explainable AI, affective computing, context awareness, intersection of AI with law, as well as human-centered AI. In 2023 he was the organizing committee chair for the ECAI 2023 conference, organized for the first time ever in Poland.